Date: 9th September 2021
Time: 10:00 (Eastern Time) / 15:00 (BST)
Using simulation examples and SmartUQ software for illustration, this webinar will cover:
- Introduction to Uncertainty
- General Machine Learning and Uncertainty Quantification Framework
- Uncertainty Quantification Methods
- Design of Experiments (DOEs)
- Predictive modeling
- Sensitivity Analysis
- Uncertainty Propagation
- Statistical Calibration
Anyone who builds complex, mission critical systems knows that real-world performance is hard to simulate and test. This is due to uncertainty. It may arise from approximations or variations in boundary and initial conditions in models, or deviations in materials, assembly, operating conditions, and wear in finished products.
Uncertainty Quantification (UQ) is a set of Machine Learning (ML) methods that puts error bands on results by incorporating real world variability and probabilistic behavior into engineering and systems analysis. UQ answers the question: what is likely to happen when the system is subjected to uncertain and variable inputs. Answering this question facilitates significant risk reduction, robust design, and greater confidence in engineering decisions. Modern UQ techniques use powerful predictive models to map the input-output relationships of the system, significantly reducing the number of simulations or tests required to get statistically defensible answers.
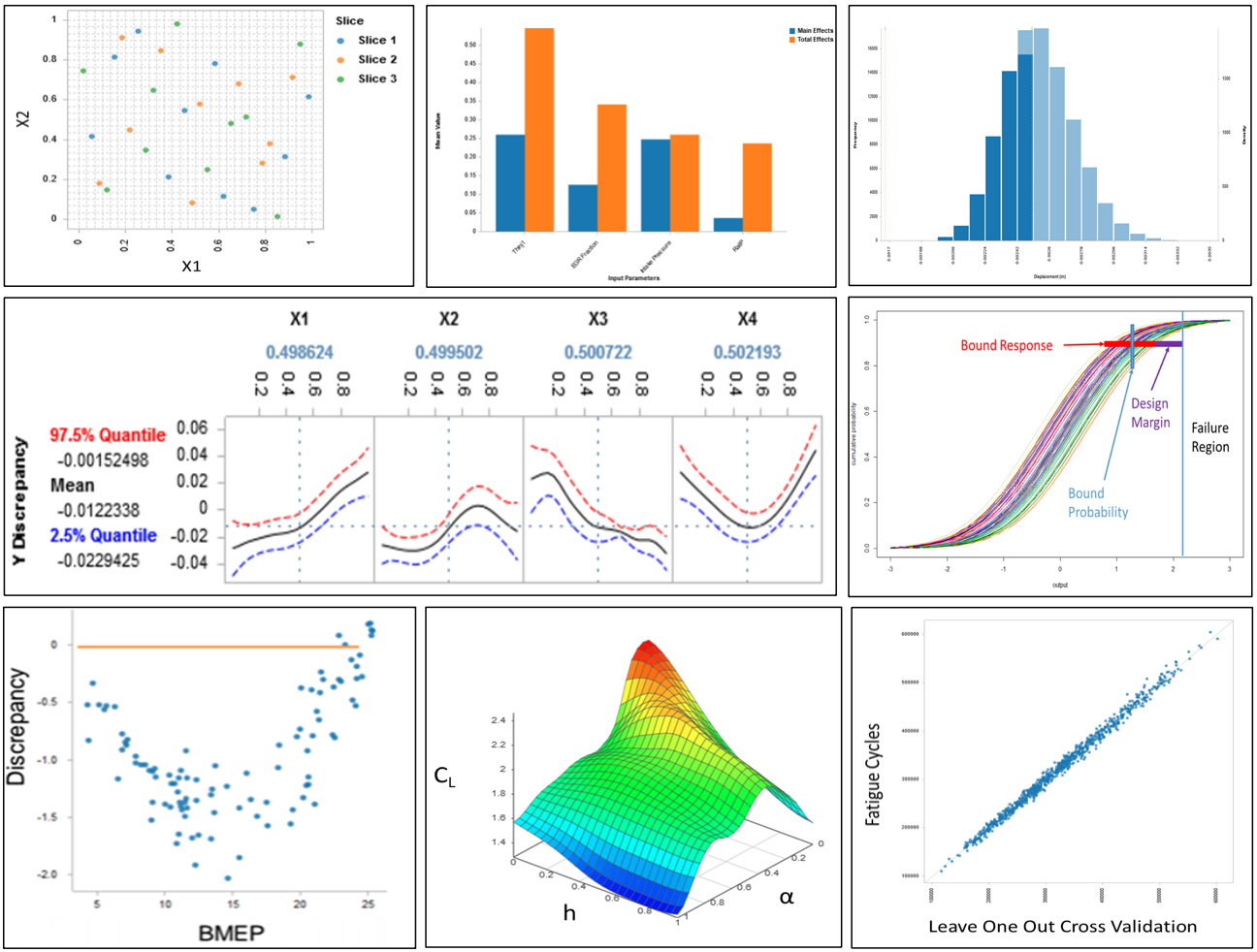